Patents on Artificial Intelligence and Machine Learning
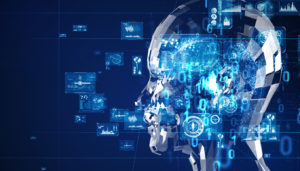
Are inventions based on artificial intelligence and machine learning patentable? As a patent attorney with an undergraduate degree in computer science and a background in artificial intelligence (AI) and machine learning (ML), I am interested in helping clients identify potentially valuable inventions that use AI or ML.
The foundations of today’s innovations in AI and ML can be traced back to work done over 30 years ago. The fundamental concepts of neural networks, genetic algorithms, natural language recognition, speech recognition, image classification, and other types of computational models, while being improved and expanded on recently, have been around for decades. From the perspective of patent law, the issues surrounding the patentability of innovations in AI and ML are also largely the same as they were many years ago. Legal analysis still begins with the same old questions: (1) is there patent-eligible subject matter, and (2) is that subject matter novel and non-obvious? Likewise, what makes a patent on AI or ML inventions valuable is generally the same as with other types of technology. We still ask: (1) are there commercially viable alternatives to the invention, (2) how close is the prior art, and (3) who and what would infringe, and how detectable would that infringement be?
Here are four basic rules one should consider when seeking to identify potentially valuable inventions based on AI or ML.
- Do not anthropomorphize
You would think from the popular media that computers are clever and can solve problems humans are incapable of solving. So much so, that many have even raised the question of whether a computer implementing AI can be an inventor! Such attribution of human capabilities to a computer is just a form of anthropomorphism and is misguided. A computer performs operations it is instructed to perform, on data it is given; it has no understanding or awareness of what it is doing or what the data represents. The computer is nothing more than a very sophisticated tool that people have chosen to apply in a way they selected, to data they chose. A lack of understanding of what a tool is doing does not make the tool an inventor; rather, it raises the question of whether using the tool in the manner it was intended is an invention. Instead, the inventors typically include those people who chose the tools to apply and how to apply them to the data they selected. - Focus on identifying the technical problem to be solved and its technical solution
The technical problem and its technical solution are the keys to identifying both patent-eligible subject matter and determining patentability. It also helps to avoid anthropomorphizing AI or ML. What can cloud this focus is the subject matter domain in which an AI or ML tool is being used, typically to solve a business problem. Focusing on the business problem solved can result in an overly abstract understanding of an invention, which is unhelpful to patent eligibility. By focusing on the technical problems and solutions, you will typically find that many AI and ML inventions are designed to solve problems relating to consistent data representation, efficient data representation for either storage, access, or processing, algorithmic efficiency, and user or system interactions, which are hidden beneath the business problems being solved. It is these kinds of technical problems that, when solved, result in improvements in computer processing and are more likely to be considered patent-eligible subject matter. - Keep in mind that some AI and ML problems were solved a long time ago
By focusing on identifying the technical problem and its technical solution, you also will be better able to identify relevant prior art. In the field of AI, it is important to recognize that today’s AI innovations are based on basic computational theory and models dating back decades. For example, my thesis work was an example of a N-nearest neighbor search; today this technique is commonly used in making suggestions on consumer websites. Back in the day, we needed custom hardware to perform this computation at a reasonable speed (1ms) on a comparatively small dataset (16000 entries). But, today, this computation is child’s play and is applied to solve a wide array of different problems (often involving large volumes of domain-specific data processed at unthinkable speed). The inventive activity typically involves figuring out how to apply a known computational model to a new problem domain, which may involve new modifications to that model, or new ways to represent data to input to the model, or new ways of interacting with the model. - Understand the market
In addition to identifying a technical problem, a technical solution, and prior art to an invention, it is also important to understand the market in which your invention will compete. The market is not merely direct competitors, but instead includes the entire ecosystem of suppliers, partners, customers, and ultimate end users. By understanding the market, it is possible to identify which activities by which entities you would want any patent to cover. You can understand the alternatives available to them, other than your invention, product, or service. Ultimately, market information helps to illuminate which invention may result in more valuable patents than others.
By following these four rules, innovators in the field of AI and ML, in any subject matter domain, should be able to identify more quickly whether they have inventions which are more likely to be patent-eligible, patentable over the prior art, and potentially valuable in their relevant market.
For questions about patents in the field of AI/ML, please contact Peter Gordon directly at pgordon@patentgc.com, or to request more information, please visit our Contact Us page.